Artificial intelligence (AI) has transformed a number of industries by providing previously unheard-of levels of automation and decision-making. But the ethical ramifications of AI decision-making are currently a widely debated subject.
The “Trolley Problem,” a thought experiment that explores the moral decisions involved in AI decision-making, is one example of an ethical scenario of this kind. Let us attempt to comprehend the Trolley Problem and investigate practical use cases that demonstrate the moral dilemmas AI presents in these fields.
What is Trolley Problem?
One well-known ethical and moral philosophy thought experiment is the Trolley Problem. It depicts a fictitious scene when someone is watching a train or trolley that is on the loose approach a set of railroad tracks. The trolley is directly approaching the group of persons who are restrained and unable to move on the rails. If someone is standing close by, they can use a lever to swerve the trolley onto another track where there is just one passenger strapped in.
The moral conundrum occurs when a person must choose between intervening to change the trolley’s tracks and possibly save many lives at the expense of one, or staying silent and letting the trolley continue on its current course, which will likely kill many people.
Why is the Trolley Problem Relevant to AI?
These days, artificial intelligence is being incorporated more and more into decision-making processes, ranging from credit evaluations and medical diagnoses to automated cars. Deep learning projects are making AI systems increasingly complicated, which increases the possibility that actions could have repercussions. For this reason, ethical considerations are necessary to guarantee the responsible and accountable application of AI.
Also Read: Choosing the Right ML Model: Your Guide
Where Does the Trolley Problem Impact AI Applications?
There are several applications where the trolley problem may occur, including but not restricted to:
- Healthcare: When allocating scarce medical resources or giving particular patient populations priority, AI-driven medical diagnosis may give rise to ethical questions.
- Finance: AI-powered credit evaluations need to be created with equity and nondiscrimination against particular demographic groups in mind.
- Autonomous Vehicles: In the event of an accident, self-driving cars will have to make snap decisions. This raises concerns about the moral factors that may influence these decisions.
How to Address the Trolley Problem in AI:
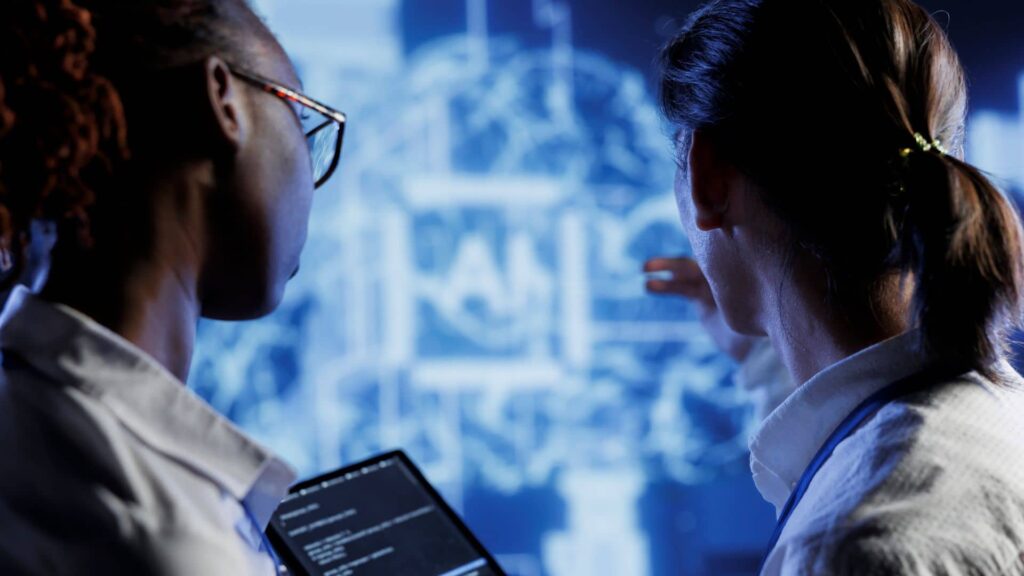
1.Transparent Decision-Making:
It is imperative to create Explainable AI (XAI) in order to address the ethical dilemmas associated with AI decision-making. XAI fosters trust and transparency by enabling enterprises to comprehend the aspects that contribute to AI-generated outcomes.
2. Bias Mitigation:
It is essential to routinely check AI systems for possible biases and take proactive steps to end prejudice. Biased results can be considerably decreased during AI model training by ensuring inclusive and diverse datasets.
3. Human-in-the-Loop Approach:
An extra level of ethical concern may be added when AI decision-making is integrated with human judgment and monitoring. Involving people in important decision-making helps reduce the possibility of unexpected outcomes.

Conclusion
In the age of AI integration, the Trolley Problem forces us to make difficult moral choices. In order to create a responsible and inclusive future, it is critical to address the ethical implications of AI as it develops.
Frequently Asked Questions (FAQs)
Q1. What are errors in machine learning?
An activity that is erroneous or faulty might be defined as an error. Error is used in machine learning to assess how well a model can predict outcomes based on both fresh and unseen data, which it has learned from. We select the machine learning model that works best for a given dataset based on our error.
Q2. What are the risks of machine learning?
These machine learning risks could include things like data biasing, overfitting, poor data quality, lack of strategy and experience, etc.
Q3. What is top 5 error in machine learning?
The percentage of times the classifier’s top five predictions do not contain the correct class is called the Top-5 error rate. To put it simply, anything that is classified using a neural network yields results that resemble probability distributions for each class.
Q4. What is overfitting in machine learning?
Unwanted machine learning behavior known as overfitting happens when a model produces correct predictions for training data but not for fresh data. Data scientists train machine learning models on known data sets before using them to make predictions.
Q5. What are the risks of AI and ML?
Nevertheless, early users of AI/ML have a higher risk of facing legal action when AI outputs incorporate copyrighted content from the internet, bias issues, lack of traceability because AI apps are “black box,” and cybersecurity and data privacy dangers.